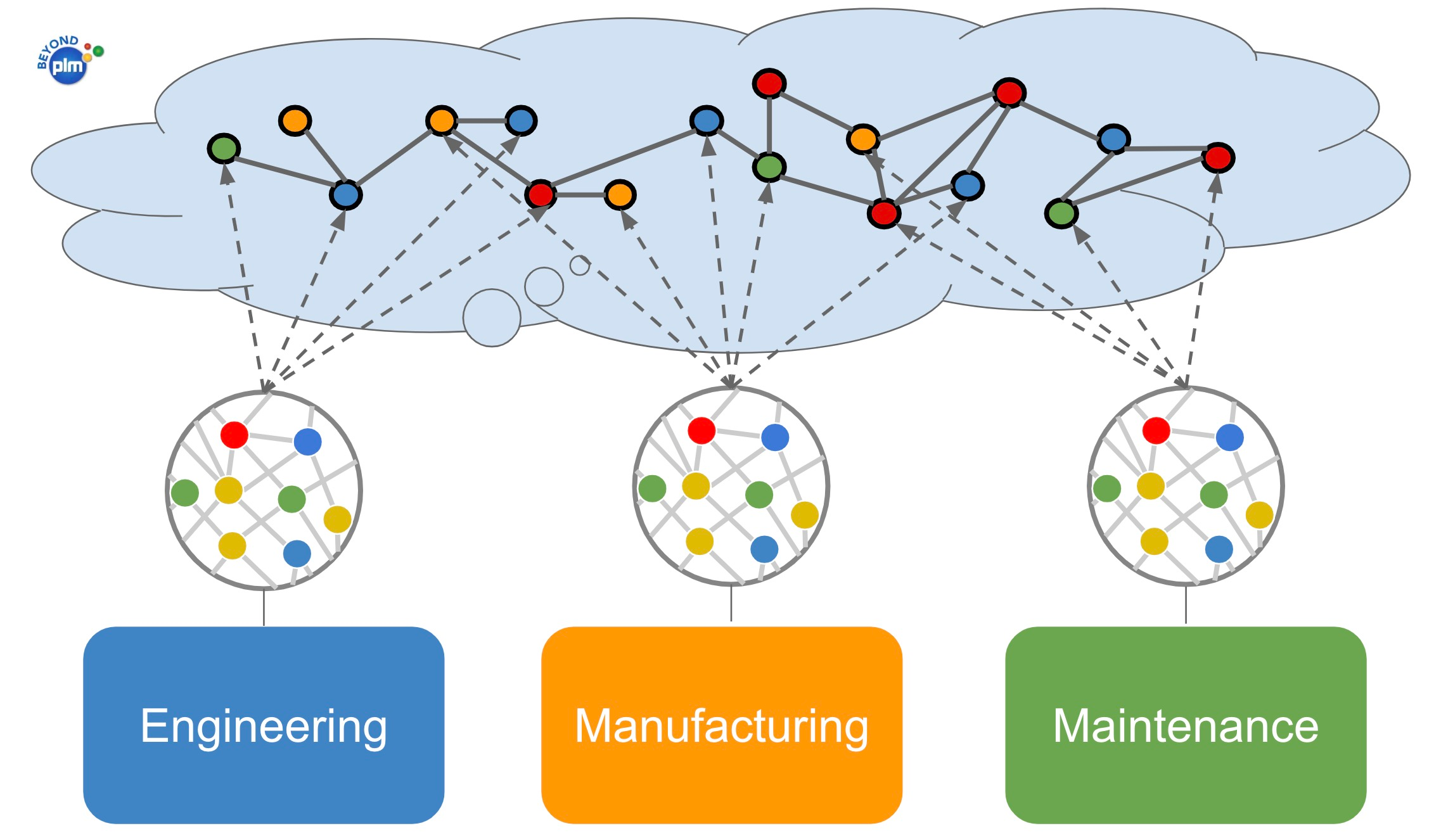
The world has shifted to a data-driven ecosystem where predictive analytics and AI automation are essential components for success in competitive markets. Just as businesses must constantly innovate new products and services to maintain market share, they must also keep abreast of emerging technologies that will help them achieve their goals. Knowledge graph technology is one such cutting-edge tool that can help manufacturing companies manage product lifecycles with greater accuracy and efficiency than ever before.
In my yesterday article, I explained the connection between digital threads, the digital web, and data that can be used for future AI software in engineering and manufacturing processes. It includes multiple disciplines such as mechanical engineering, electrical engineering, manufacturing processes, supply chain management, and many others. Today, I want to continue the conversation and talk about the importance of knowledge graphs for the future of product lifecycle management software.
What is wrong with existing PLM platforms?
All mindshare PLM platforms’ architecture was built 20+ years ago and mostly satisfies the needs of mechanical engineers. The newest out of them is Aras and the oldest are Teamcenter and Windchill. Dassault Systemes 3DX platform is a major upgrade of the acquired 15+ years ago MatrixOne platform. Regardless of how sophisticated and optimized these platforms are, all of them are built on SQL database architecture that has limited semantic capabilities and scale to satisfy the needs of growing digital environments. All of them are a single tenant and their cloud (SaaS) roadmaps are basically focusing on how to get existing platforms installed (hosted) and automated (DevOps) using cloud platforms such as AWS, Azure, GCP, and others.
All these platforms are vital for existing customers and therefore won’t die for the foreseeable future. At the same time, these platforms have limited digital modeling capabilities, capabilities to manage interdisciplinary engineering needs, collaborative engineering tasks, query expressiveness, large-scale queries, and flexibility to satisfy the business needs and expectations of modern manufacturing business processes.
Why knowledge graphs?
A knowledge graph is a way of organizing and representing data that helps to create a comprehensive and interconnected understanding of a subject. It is essentially a structured network of information that can be used to make informed decisions and gain insights into complex systems.
In engineering and manufacturing, knowledge graphs can be used to represent a wide variety of information, including design specifications, product attributes, manufacturing processes, and supply chain logistics. By creating a knowledge graph, engineers and manufacturing professionals can better understand the relationships between different components of a system and make informed decisions about how to optimize production processes, reduce costs, and improve product quality.
Knowledge graph benefits
The knowledge graph is a clever invention how to increase semantic metadata management and improve the data management capabilities and scale of PLM systems. It is also an opportunity to capture and prepare the data that can be later used for machine learning algorithms. It is hence a clever invention of modern data management to help upscale existing PLM platforms to manage not only mechanical engineering disciplines but also to scale to support multi-disciplinary systems with up-to-date information that elsewhere cannot be achieved. This is where the digital thread begins and systems will be capable to build a knowledge base for a broad scope of engineering and manufacturing processes for product lifecycle management systems.
One of the key benefits of using a knowledge graph in engineering and manufacturing is that it can help to identify areas where improvements can be made. By analyzing the relationships between different components of a system, engineers and manufacturing professionals can identify bottlenecks in the production process, opportunities to reduce waste and potential areas for innovation. This can lead to significant improvements in efficiency and productivity, ultimately resulting in cost savings and improved product quality.
Another important use of knowledge graphs in engineering and manufacturing is in the design and development of new products. By using a knowledge graph to represent the different components of a product and their relationships, engineers can better understand how changes to one component will affect the overall system. This can help to optimize the design process and ensure that the final product meets all of the necessary specifications.
Last, but not least, knowledge graph-based PLM systems are capable to provide analysis and impact of changes with a much higher level of comprehensiveness and lead to improvement in change management processes, change impact, and cost analysis. The computer science behind graph algorithms, operation with diverse data, and artificial intelligence will allow bringing PLM to the next level that is not achievable using traditional PLM platforms.
What is my conclusion?
In the era of digital transformation, the importance of knowledge graphs for Product Lifecycle Management (PLM) cannot be overstated. By providing a structured way to organize and analyze complex data, knowledge graphs can help to improve efficiency, reduce costs, and improve product quality. Legacy PLM platforms are limited in their semantic capabilities, and query scale as well as the ability to scale organizationally across multiple companies and supply chains. Demand from engineering disciplines and computer engineering to improve a manufacturing process will trigger interest in AI and will drive even higher interest in new capabilities of knowledge graph and graph databases. 4th industrial Revolution will change manufacturing, mass production, and a large number of engineering disciplines. As the field of engineering and manufacturing continues to evolve, it is likely that the use of knowledge graphs will become even more widespread, helping to drive innovation and progress in these critical industries.
Just my thoughts…
Best, Oleg
Disclaimer: I’m co-founder and CEO of OpenBOM developing a digital-thread platform with cloud-native PDM & PLM capabilities to manage product data lifecycle and connect manufacturers, construction companies, and their supply chain networks. My opinion can be unintentionally biased.