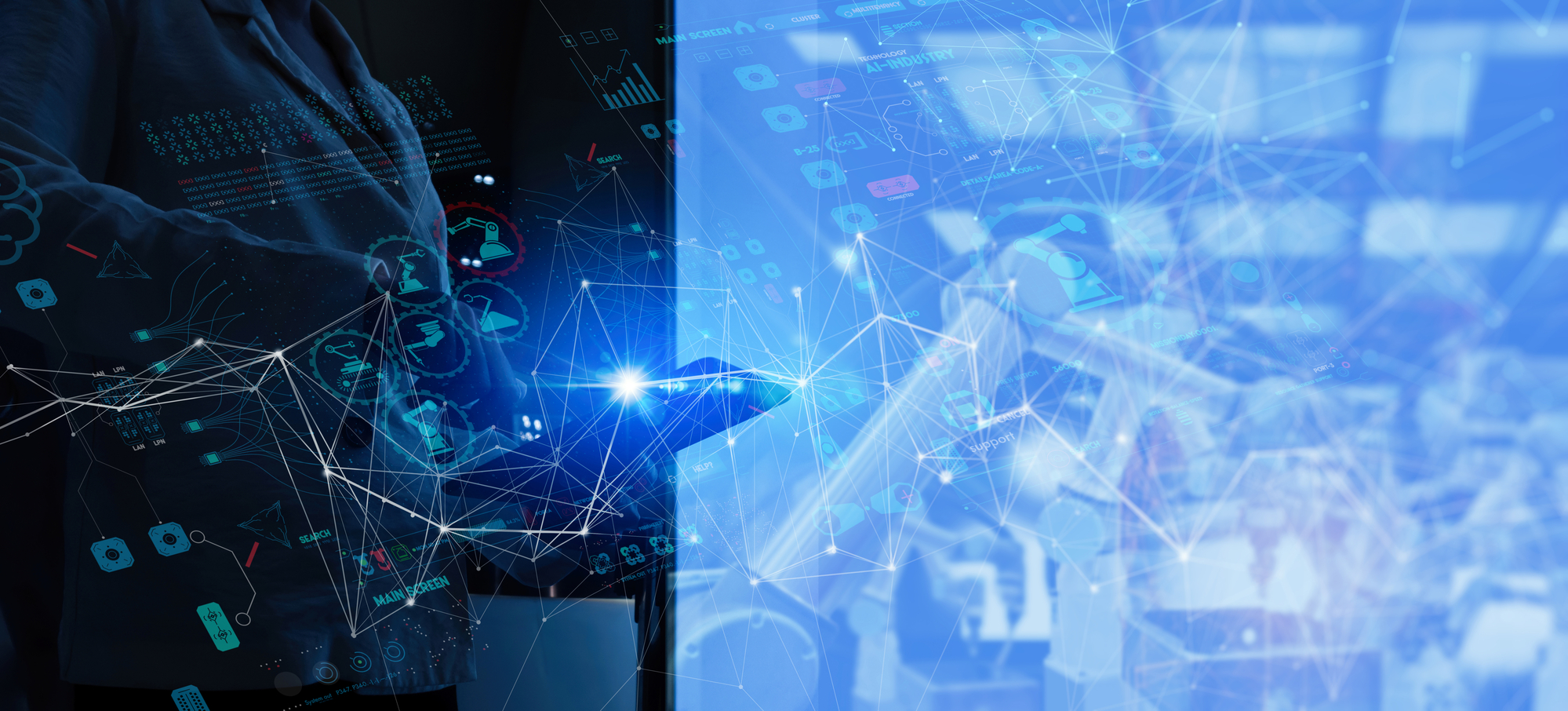
In today’s fast-paced business world, it is important for companies to keep up with the latest technology and tools to stay competitive. Product Lifecycle Management (PLM) is one such tool that has gained significant attention. CIMdata Industry Forum is one of the places where you can get an annual injection of PLM industry news and analytics.
I’m continuing to digest the information from the last week’s CIMdata 2023 industry and market forum I attened last week in Ann Arbor, MI. If you missed my previous article 5 Important Things about the State of PLM, check it out. The main theme of the forum was Digital Thread and today I want to talk about some elements of data infrastructure that quickly becoming very important for digital transformation and future PLM development, In the picture below you can see the way CIMdata presents Digital Thread – a communication framework to connect data flow and integrated view.
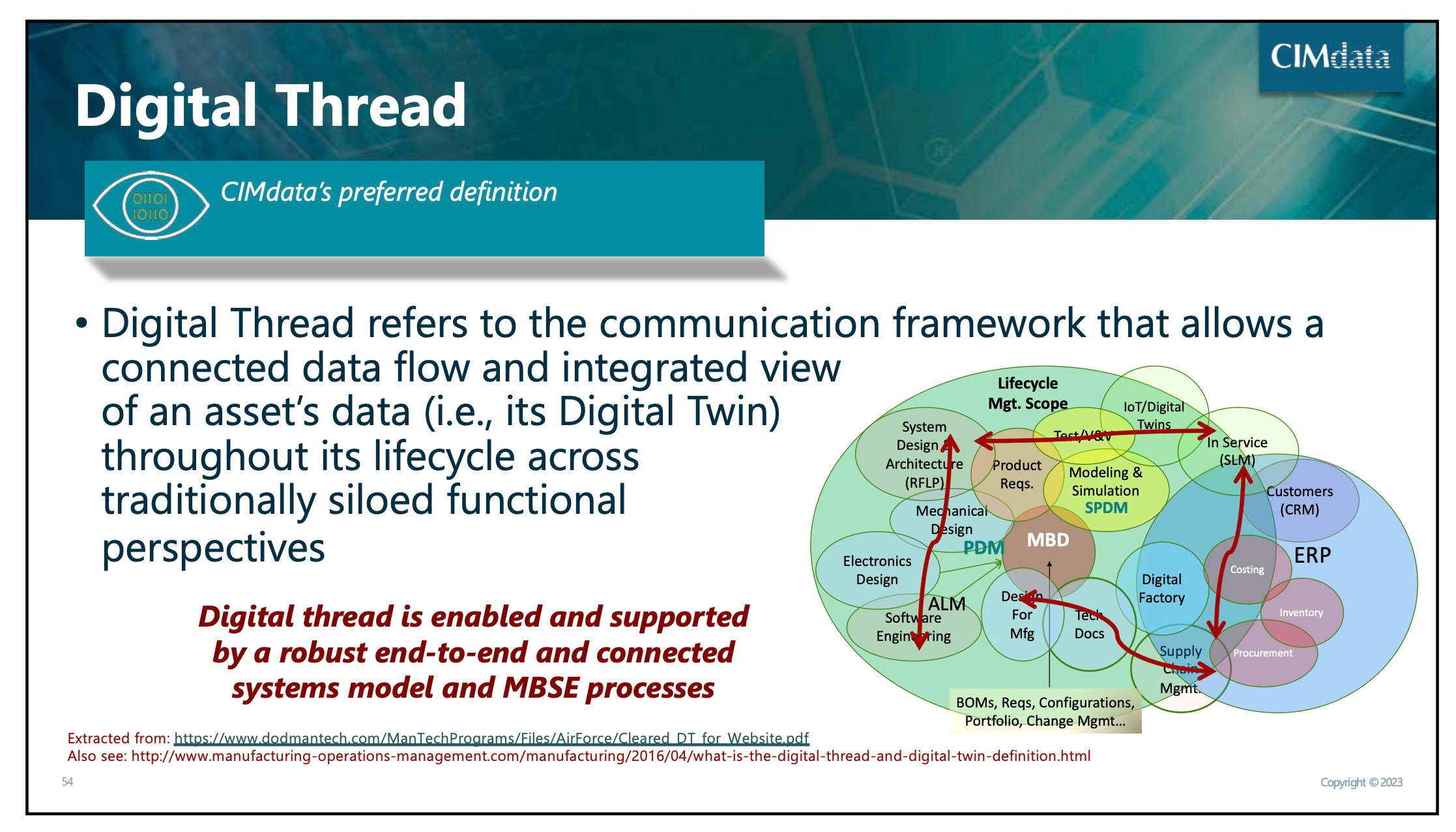
From my experience, PLM implementations can successfully build information flow in the way of building integrations and “pumping data” between multiple systems based on some basic logic. Those are usually strategic projects with substantial investment in tools and business scenarios. The quintessential example of this type of integration is the Siemens Teamcenter-SAP integration partnership. If you haven’t seen it, watch the video to see what is about.
PLM: From Digital Thread to Digital Web
The digital thread represents the timeline sequence of product information. From the lifecycle standpoint, you can think about it as a sequence of BOMs (structures) such as design, engineering, manufacturing, and service. This is one perspective. A different dimension is to get a view of all derivative dependencies. This is what CIMdata calls a Digital Web. Both have direct relationships to the information flow.
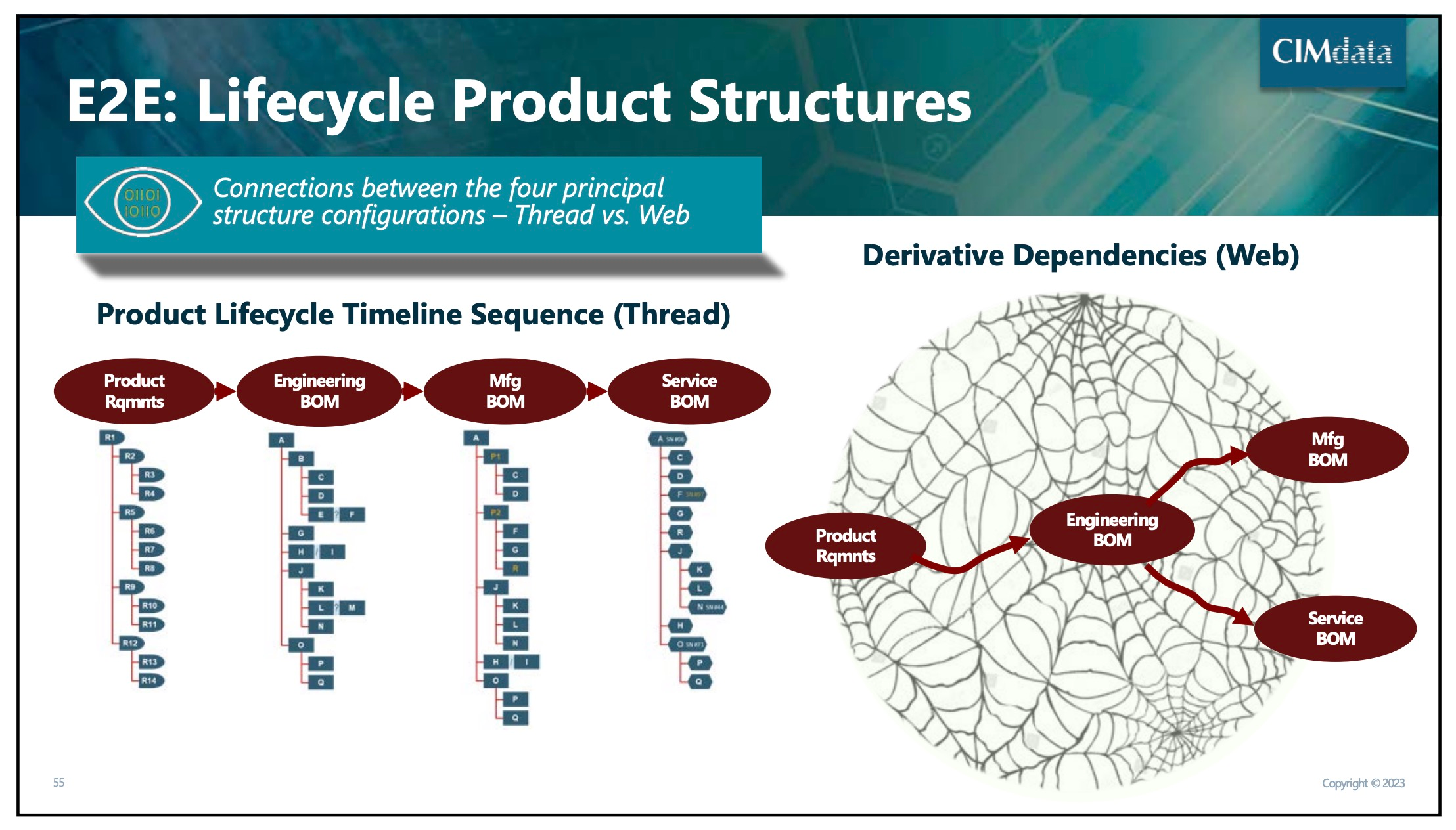
And this is what the full picture of a product lifecycle management digital web can look like (in the version of CIMdata). The digital thread and digital web are two related concepts that have emerged along with PLM.
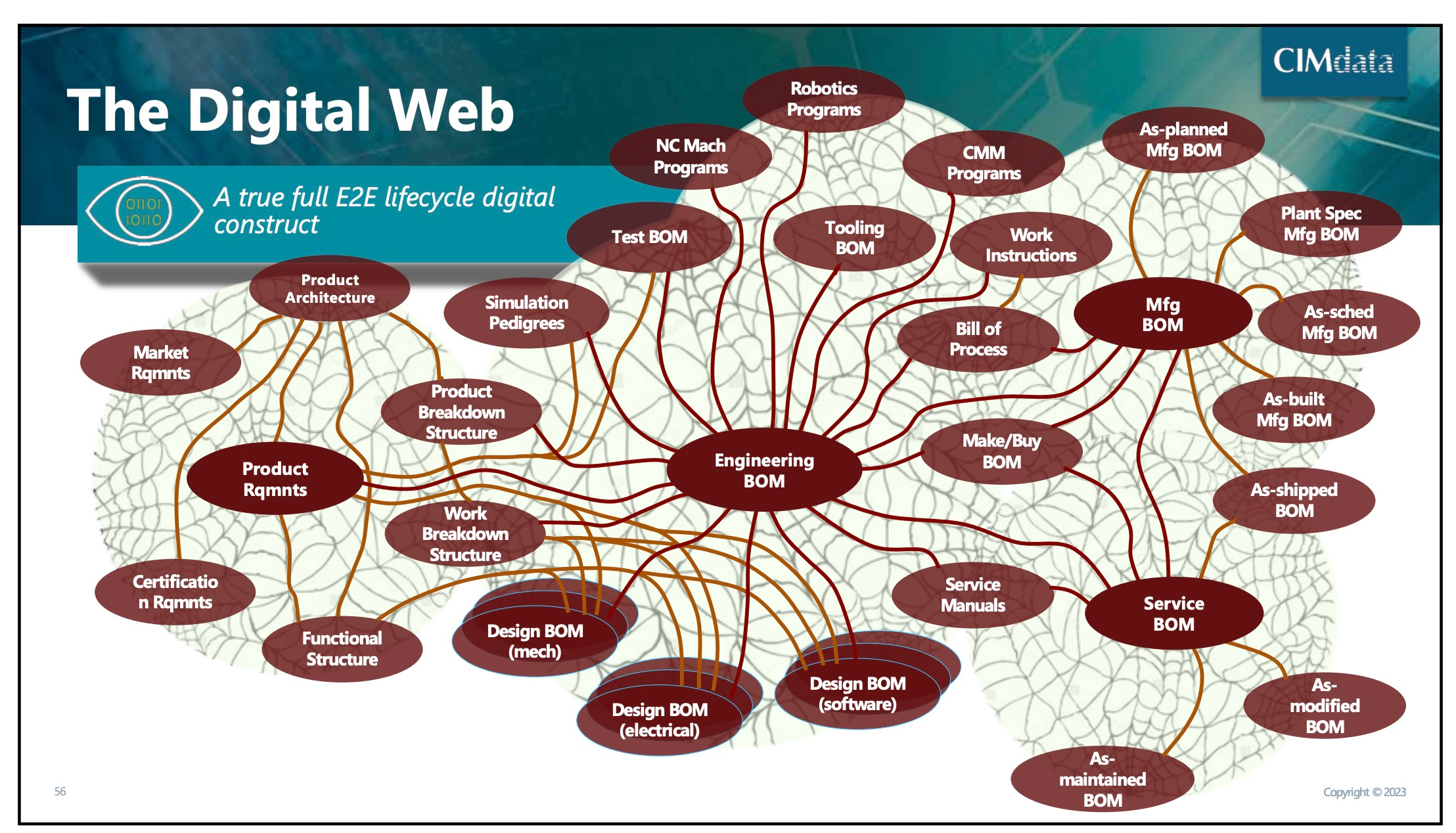
The digital thread is a concept that is closely related to PLM. It refers to the digital representation of a product throughout its entire lifecycle. The digital thread connects all the data related to a product, from its initial design to its final retirement. By creating a digital thread, companies can improve communication and collaboration between different departments and stakeholders. This can help reduce errors and improve product quality.
The digital web is another related concept that builds on the digital thread. It refers to the ecosystem of digital data that surrounds a product. This includes data from suppliers, customers, and other stakeholders. By creating a digital web, companies can improve supply chain visibility and traceability, which can help reduce costs and improve product quality.
Together, PLM, the digital thread, and the digital web form a powerful ecosystem of tools and concepts that can help companies manage the entire lifecycle of a product. By leveraging these tools, companies can improve communication and collaboration, reduce costs, and improve product quality. In today’s competitive business environment, these tools are essential for companies that want to stay ahead of the curve.
From Digital Web To Knowledge Graph
A knowledge graph is a powerful tool that helps to organize and connect complex data and information. It is a network of nodes (or data points) that are connected by edges (or relationships) that represent the connections between them. This structure allows for easy navigation and analysis of data, as well as the ability to uncover new insights and connections that may not have been apparent before. Google Knowledge Graph is a great example of how information can be organized and used for practical goals. Check a Google article from 10 years ago explaining about knowledge graph –things, not strings.
In the context of PLM, a knowledge graph can be used to represent and connect all of the data and information related to a product, including design files, manufacturing instructions, quality control data, service records, and more. By organizing this data in a knowledge graph, businesses can gain a holistic view of the product throughout its entire lifecycle, allowing for better decision-making and more efficient processes.
The field of building knowledge graphs and semantic layers for manufacturing is just at the beginning. Companies working on semantic technologies were investing in this field. Here is an example I picked in the Ontotext article:

Here is a very high-level example of the knowledge graph vision I shared in my article a few years ago in my article – Manufacturing Knowledge Graph, Why It Matters and Why It Is Important For PLM.

To sum up, a knowledge graph is a structured representation of knowledge that captures relationships between entities and concepts. It is essentially a network of nodes (representing entities) connected by edges (representing relationships) and attributes (representing properties). Knowledge graphs are used to organize and represent data in a way that can be easily queried, searched, and analyzed. They can be thought of as a kind of semantic database that captures the meaning of data and relationships between entities.
GPT and Knowledge graphs For More Accurate Queries
In recent years, GPT (Generative Pre-trained Transformer) models have become a popular choice for natural languages processing tasks, such as language translation, text summarization, and question-answering. These models have achieved state-of-the-art results, thanks to their ability to learn from large amounts of text data using unsupervised learning techniques. However, the performance of GPT models is directly dependent on the data you loaded and can be further improved by incorporating knowledge graphs.
If you’ve been using ChatGPT recently, you probably generated some level of concern about the accuracy of the answers. The so-called “hallucinations” of the GPT model are well-known behavior. After all, statistically, the words make sense, but the data can be not accurate and you can be concerned to use this data for missing critical tasks.
Here is where I can see some future interest in mixing both models to provide accurate results and enrich both models. Both way benefits are actually interesting – the graph model can help to improve disambiguation in the text, but on the other side, the Knowledge graph can obtain correct results.
Here is an interesting example in the article transforming queries using GPT3 and then using the outcome in Cypher queries – English to Cypher with GPT-3 in Doctor.ai. Although not an example from the manufacturing industry, it can give you an idea of how this algorithm can potentially work together allowing you, for example, to build a BOM for a new model of electric bike training on specific company data and use a knowledge graph created from specific customer data. It can be an exciting opportunity for the future development of digital tools to solve new product development, supply chain management, predictive maintenance, and other business processes. Building it requires a diverse data set, enriched with semantic metadata and modern machine learning algorithms.
Conclusion
The future of the PLM roadmap will be different from what we’ve seen for the last 20 years. New business models and modern business processes require new tools. You cannot build future products with yesterday’s tools. Having up-to-date information is not enough anymore. There is a need and opportunity to use digital thread, and digital web data to build knowledge graphs and use it together with machine learning and GPT technologies. New tools will be using modern data management technologies and machine learning to help engineers and manufacturing companies to build great new products.
Best, Oleg
Disclaimer: I’m co-founder and CEO of OpenBOM developing a digital-thread platform with cloud-native PDM & PLM capabilities to manage product data lifecycle and connect manufacturers, construction companies, and their supply chain networks. My opinion can be unintentionally biased.