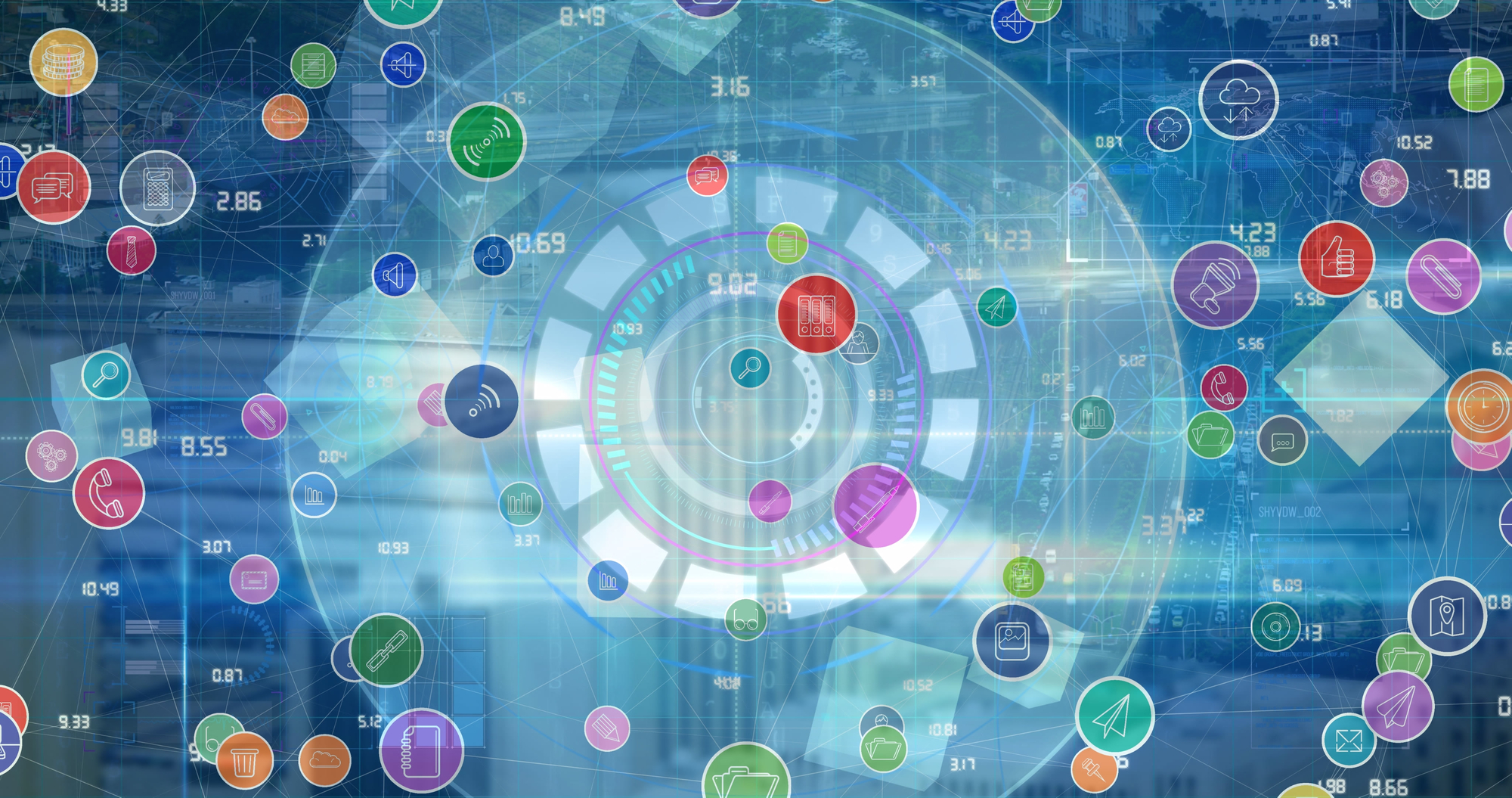
In today’s rapidly evolving landscape of data management and artificial intelligence (AI), the relationship between the two is becoming increasingly apparent. It is hard to view both domains AI and data management as separate entities. If I want to think about complex data management problems, it would be a mistake to see them separate. The previous generation of data management systems was relying on a specific database type (eg. RDBMS) and developed applications while managing data using tools provided by a database. All mindshare PLM vendors today using this architecture for their systems such as Aras, Dassault 3DEXPERIENCE, Oracle, SAP, Teamcenter, and Windchill (with the exception of SAP for HANA) However, based on the but the majority of SAP customers are using Oracle.
The things are going to change and I can see many indications of developing new data management architectures capable to use multiple databases operating together and, most importantly, integrated and coordinated together, which brings up a question about what trajectory PLM data management systems and architecture will go.
My attention caught by a publication by Tony Seale speaking about how he can see connections between different data management systems and data models.
Here is an interesting passage:
In an enterprise context, AI models unlock their true potential not merely through the vast quantities of information they process, but when that information is relevant, connected, cleaned, structured, and enriched with semantics—all tasks that lie at the heart of data management expertise. Yet, the reverse is equally true: our data strategies gain direction and sophistication when guided by the insights and capabilities AI brings to the table. Moreover, AI can help you connect, clean, structure, and enrich your data with semantic metadata.
No longer can we view AI and data management as separate entities; instead, they should be seen as complementary forces driving innovation and efficiency within enterprises. At the heart of this paradigm shift lies the understanding that AI thrives on relevant, connected, structured, and enriched data – tasks that are central to the expertise of data management. Conversely, data strategies reach new heights when informed by the insights and capabilities AI brings to the table. This mutual dependency underscores the need for a cohesive approach to AI and data management within organizations.
Challenges of PLM and Structured Data Modeling
The modern knowledge graph modeling experience made me think about current trajectory and implication of these technologies on existing enterprise PLM systems. PLM, which represents the process of entire lifecycle of product from early requirements through disposal demands a holistic approach of comprehensive data management systems. At the same time, what we have is 20+ years old data modelers using SQL databases foundation.
Traditionally, PLM data modeling has focused on organizing explicit data within structured databases. While this approach has served its purpose, the evolving landscape demands a more nuanced and interconnected framework. The main thesis, drawn from contemporary insights, suggests that PLM data modeling must evolve to product knowledge graphs from traditional datasets.
Connecting existing PLM systems to modern data architectures including knowledge graphs and AI foundation is a strategy that can expand the richness of data models and provides new capabilities to integrate existing data into KGs and AI data principles allowing to capture more information semantics from existing design (CAD) documents, design specifications, requirements and other data silos. Effectively harnessing this data is key to driving innovation, improving product quality, and enhancing competitiveness.
Knowledge graphs – A bridge towards PLM intelligence?
Knowledge graphs offer a disciplined approach to structuring data, making it not only accessible but also readily usable for AI applications. By representing data as a network of interconnected entities and relationships, knowledge graphs facilitate a deeper understanding of complex systems, enabling more informed decision-making and predictive capabilities.
The synergy between AI, knowledge graphs, and PLM is evident in their shared objective of transforming raw data into actionable insights. AI algorithms can leverage the rich semantics encoded within knowledge graphs to infer relationships, identify patterns, and make intelligent recommendations. In turn, knowledge graphs benefit from AI-driven techniques for data cleansing, enrichment, and semantic annotation, ensuring the integrity and relevance of the underlying data.
Check some of my earlier articles about knowledge graphs and product models. I can see how graph bases product models will become a new source of information modeling to create a foundation for AI in the future.
The importance of knowledge graph for future PLM platforms
How to build product model for digital thread
3 benefits of using graph based digital thread product model
PLM Evolution towards Knowledge graphs
The evolution towards knowledge graphs in PLM data modeling addresses the important challenge of “garbage in, garbage out.” Manufacturing companies are drowning in the data. Existing PLM systems are barely scratching a surface of data management mostly focusing on management of CAD data (files), engineering release and change management processes. The large amount of data is distributed between siloed databases, spreadsheets of mega-size and legacy systems.
To get the information from these systems organized, and semantically connected is a big deal. And this is what effective data management using knowledge graph based systems can do. It can bring relevant data and to create a data architecture to support data governance and data analysis. Data stored in multiple data management systems combined with data pipelines organized between existing enterprise PLM system, graph databases to build knowledge graph and other data management services will help product lifecycle management to collect organization’s data into a combined data set that can be used to feed AI systems.
By structuring data in a meaningful way and leveraging AI-driven approaches for data validation and cleansing, organizations can mitigate the risks associated with poor data quality, ultimately enhancing the efficacy of their AI initiatives.
What is my conclusion?
The convergence of PLM data, knowledge graphs and AI brings a new era of innovation and efficiency in product lifecycle management. By embracing this integrated approach, organizations can unlock the full potential of their data assets, drive continuous improvement, and maintain a competitive edge in an increasingly dynamic marketplace.
The proposed architecture will allow to leverage majority of enterprise PLM assets with the large history of engineering data and enrich it using modern technologies. The journey from data to product knowledge graphs empowered by AI represents not just a technological advancement, but a strategic imperative for businesses seeking to thrive in the digital age. Just my thoughts…
Best, Oleg
Disclaimer: I’m co-founder and CEO of OpenBOM developing a digital-thread platform with cloud-native PDM & PLM capabilities to manage product data lifecycle and connect manufacturers, construction companies, and their supply chain networks. My opinion can be unintentionally biased.