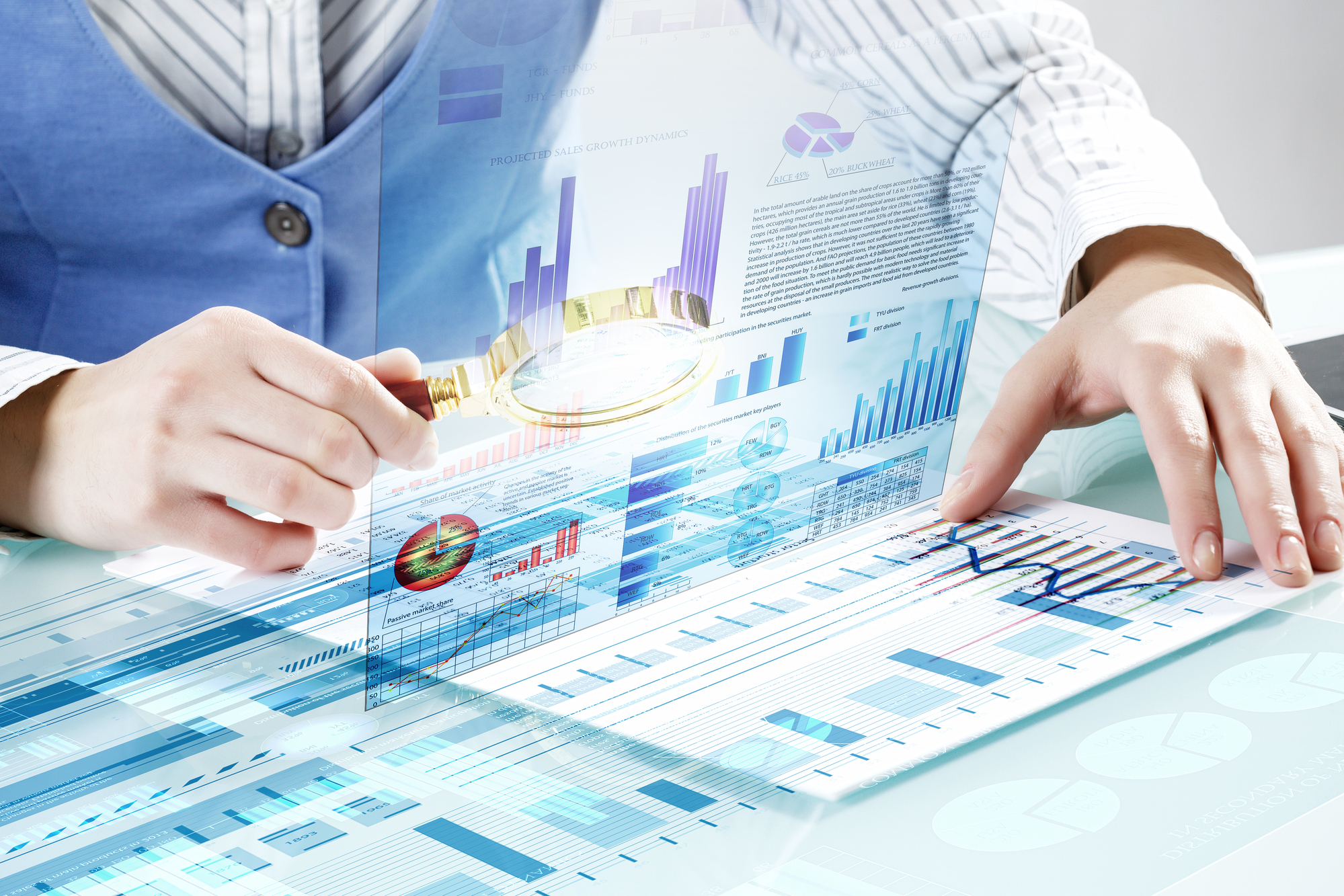
In today’s increasingly digitized business environment, organizations are producing huge amounts of data that can be leveraged to drive strategic decisions and enhance operational efficiency. As the role of data in decision-making becomes even more important, managing and accessing this data effectively becomes critical. Those two things are probably not surprising you at all. PLM companies are in full swing of actions to support digital transformation strategies, digital threads, and many other features. These strategies are supposed to deliver capabilities to use data in business processes and data analysis. The goal of companies is to have fully digital business processes to support their digital transformation strategy and efficient supply chain management.
How it can be done in practice? Product lifecycle management (PLM) data is super complex and not really located in a single database (eg. PLM, ERP, CRM) and also spanning between multiple departments, geographies, and even multiple PLM systems.
My attention was caught by Michael Finnociarro’s article – Demystifying Data Discovery and Enterprise Search in Product Lifecycle Management – Navigating the Product Data Jungle which wrote about search and data discovery tools in the PLM world.
One of Michael’s conclusions is about what market needs triggered my special interest:
What the market needs is a strong Enterprise Search tool that gathers all the product information to feed both the master data management (either in an MDM like Stibo or an ERP like SAP or Dynamics) and the PLM with clean, relevant data for decision-making and design innovation. The Data Discovery then can be tacked on to get behavioral information into the process to see how customers react, how the product behaves, what the what-if simulations say, etc. Today’s Enterprise Search engines do not focus on technical data which represents a massive opportunity for vendors in the manufacturing space.
For the last 20 years, search became a ubiquitous tool that is used everywhere online and inside different applications. For many users today, search is an obvious thing that exists in any application and tool, so we even stopped thinking about it. However, when you start touching it more in-depth, you can quickly realize that search is actually the beginning in a much deeper conversation about data availability in organizations.
Product Lifecycle Management (PLM) Data
PLM represents an information-driven approach that manages a product’s entire lifecycle, from conception to disposal. The data related to a product in the PLM system encapsulates all the information associated with the requirements, CAD design, manufacturing, services, and end-of-life of the product. This can include CAD drawings, manufacturing process details, service history, and feedback data.
The data in a PLM system is invaluable. It helps to streamline the product development process, improve efficiency, reduce errors, and ensure regulatory compliance. However, as the volume of data grows and becomes more complex, it can become increasingly challenging for organizations to manage and access it effectively.
The Challenge of Data Access and Discovery
Data access and discovery in PLM can be challenging due to several reasons. First, the data is usually stored in a variety of different formats and locations. This can make it difficult to locate specific pieces of information and can lead to data silos. Additionally, the complexity and volume of the data can make it difficult to comprehend and analyze, resulting in missed opportunities for optimization and improvement.
Getting back to Michael’s demand to have a searchable data set capable to traverse the entire product data regardless of location, and system and also to absorb a collective set of rules about how to make a specific data set visible or invisible to a specific user, organization, and process.
It is tough from both standpoint – (1) organizational (there are so many aspects of the data discovery process that needs to be followed) access, and (2) technological (most applications need to produce a full index to get access to data with the large scale, it is a very complex problem.
The last thing to mention is to have connectivity between multiple systems to get access to the data. Most enterprises are using multiple systems, SaaS applications, data centers, and many other tools.
Enterprise Search: A Solution to PLM Data Complexity?
Enterprise Search can provide a solution to these challenges. It is a technology that allows users to search for information across multiple enterprise-level data sources in a seamless manner. This can include databases, file systems, email, intranets, and other data sources.
When applied to PLM, Enterprise Search allows users to quickly locate and access the information they need, regardless of where it is stored or the format it is in. This can significantly reduce the time and effort required to find information, and can also help to break down data silos.
In addition, many Enterprise Search solutions incorporate machine learning and artificial intelligence technologies. These can be used to analyze and categorize the data, making it easier to understand and draw insights from. This can provide a major advantage in PLM, where the ability to quickly analyze and act on data can be a key factor in maintaining a competitive edge.
While some PLM vendors are embedding search tools as part of their portfolio, I wonder how much of “search” is still available offline in the old legacy systems. To get access to that data is a real barrier for a successful digital transformation.
Data Discovery: A Step Further
Google was teaching us for the last 20+ years how search should work. Everyone got it. But what good for ranking in Google won’t apply for Enterprise Search? But PLM data is complex. Sometimes you don’t know what you don’t know and this is an opportunity to provide another way to traverse the data – data discovery.
Data discovery takes things a step further. It is a process that allows users to uncover patterns, correlations, and anomalies in the data without a predefined hypothesis or query.
In PLM, Data Discovery can be invaluable. It can be done in a simple way (producing regular user interface for data), but it can also do in a variety of forms and functions- graphical networks. large diagrams and other ways to slice and dice this data.
It can help to identify potential issues or opportunities that may have been overlooked and can provide a deeper understanding of the product lifecycle. By combining Enterprise Search with Data Discovery, organizations can not only access and understand their data but can also proactively explore and gain insights from it.
Beyond Search and Discovery: Knowledge Graph and AI
Utilizing Knowledge Graphs and AI goes beyond traditional search and discovery by constructing a semantic network of information, where nodes represent entities and edges represent the relationships between them. This not only allows for a more comprehensive understanding of data but also enables AI to draw contextual and relational conclusions, boosting the efficiency of information retrieval, enhancing the user experience with personalization, and opening up avenues for new research and innovation in various domains including healthcare, e-commerce, and social networking.
Check some of my recent articles about this topic: The importance of knowledge graphs for future PLM platforms. Knowledge graphs are very fast becoming a tool and tech to complement existing AI solutions.
The real opportunity in my view, lies in the ability of knowledge graph to connect data coming from multiple places and organize semantic navigation and discovery of the data. The graph model is key to this technology to allow scalable navigation and abstraction levels to capture all the data. Check my earlier article – Why graph knowledge model is a future of manufacturing and production lifecycle.
What is my conclusion?
In the era of digital normality, data is at the heart of business operations. Managing and understanding this data is crucial for driving strategic decisions and maintaining competitiveness. In PLM, this is particularly true due to the complexity and volume of the data involved.
By leveraging Enterprise Search and Data Discovery, organizations can more effectively manage and gain insights from their PLM data. This can help to streamline the product development process, improve efficiency, and uncover new opportunities for optimization and improvement. Ultimately, these technologies are more than just tools – they are essential components in navigating digital normality and driving business success.
However, the complexity of data in PLM and in manufacturing organizations is overwhelmingly large. While search and navigation tools are important, as an organization, you need to think about how to retain the strategy for getting to a holistic data set. This is where I can see the future of the knowledge graph laid down in the enterprise.
Just my thoughts…
Best, Oleg
Disclaimer: I’m co-founder and CEO of OpenBOM developing a digital thread platform including PDM/PLM and ERP capabilities that manages product data and connects manufacturers, construction companies, and their supply chain networks. My opinion can be unintentionally biased.