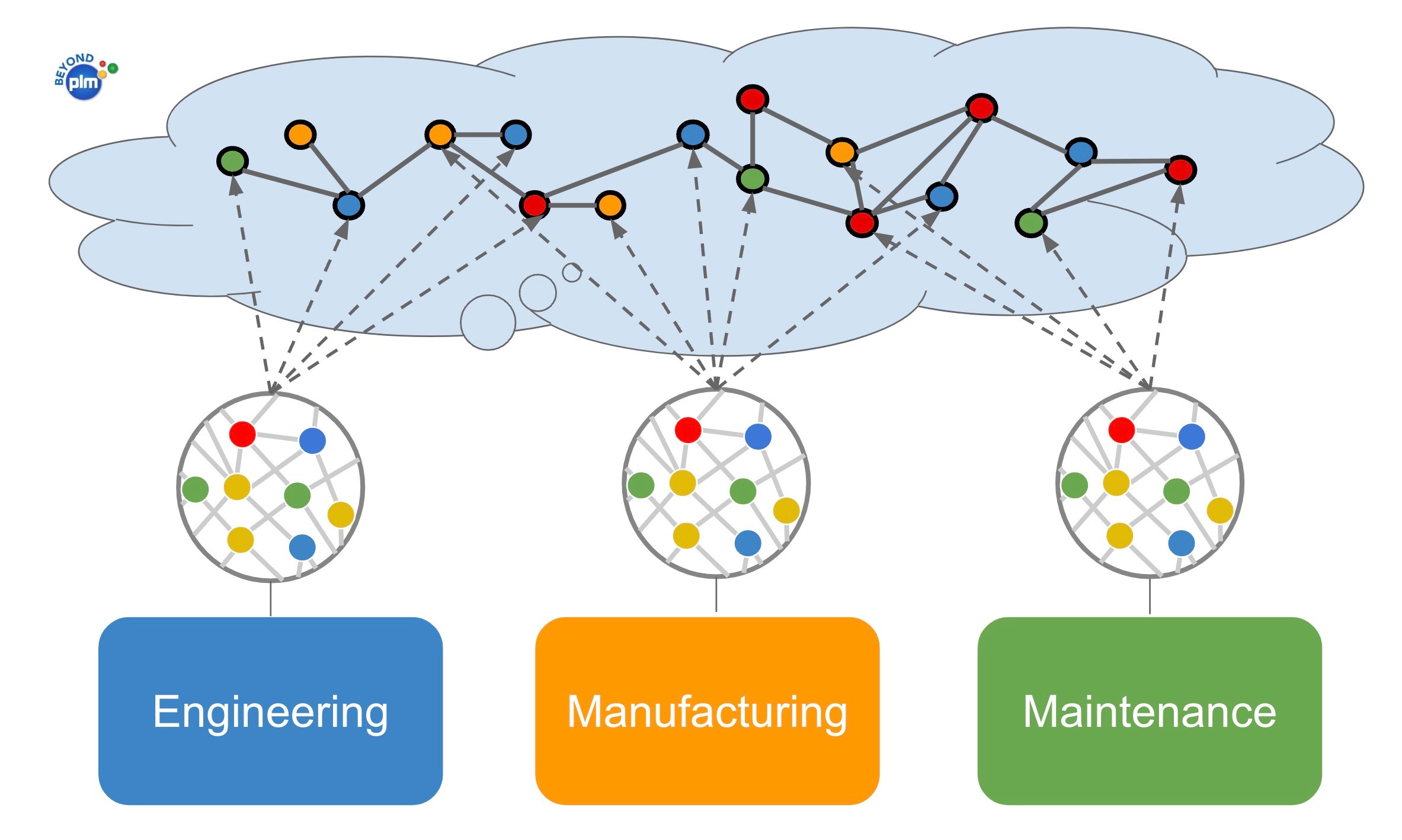
The complexity of products has grown enormously for the last decade. The growing number of manufacturing companies are moving their business models from selling product to selling services (and renting the equipment), modern products are blend of mechanical, electronic, and software components, many products are require maintenance, also products can change their configurations and feature using software components. This is just a few examples. I’m sure you can bring more. With this evolution comes a cascade of challenges, particularly in managing the vast array of data layers throughout a product’s lifecycle. What it means is that companies need to maintain a digital replica of the product (lifecycle digital twin) that represents the product as it goes through the its lifecycle stages after being shipped to the customer. In the past, the number of long lifecycle product was limited to aerospace and defense. These days, the number of products that requires after manufacturing data management, maintenance and support record is growing. Add to this group complex industrial building and such as data centers, and many other industrial installation. From its inception in engineering to its ongoing maintenance, each phase demands meticulous attention to detail and a robust data management strategy.
The Crucial Importance of Multilayered Data
In the modern manufacturing landscape, the complexity of products has skyrocketed. Take, for instance, a complex industrial machinery or robotics system: it’s not just a machine with moving parts anymore. It’s a sophisticated combination of mechanical systems, intricate electronics, and complex software algorithms. As products become increasingly multifaceted, preserving multiple data layers becomes imperative.
Consider the process of maintenance and software upgrades for an equipment manufacturer. The task goes far beyond simple repairs; it involves navigating through layers of complex data, from mechanical schematics to software components. Without a comprehensive approach to managing these data layers, companies risk inefficiencies, errors, and even safety hazards. The article by Conrad M. BittesBeyond the realm of digitalization gives some interesting examples related to the recent Boeing incident with door maintenance plug. Here is the passage:
13 March 2024, a letter (Ref.1) is issued from the NTSB (the National Transportation Safety Board in the US) stating that: “We still do not know who performed the work to open, reinstall and close the door plug on the accident aircraft. Boeing has informed us that they are unable to find the records documenting this work…” “The absence of those records will complicate the NTSB’s investigation moving forward”.
In the struggles to understand what happened on the Alaska Airlines Boeing 737, where a piece of the fuselage, the so-called “door plug”, flew off after some minutes from takeoff, a new problem appeared: Boeing is unable to provide associated documentation and not even able to identify the personnel that conducted the assembly work.
The picture below speaks better than anything else. There is a need to have a data management systems capable to maintain the complexity of multiple data layers. In other words – multiple BOM types to represent the data in each independently maintained data structure with all items and related documentation and historical records of what is happening with the products.

The Dominance of PLM in Engineering Release and Change Management
Traditionally, Product Lifecycle Management (PLM) systems have primarily focused on engineering release and change management. These systems excel in streamlining the design process, ensuring version control, and managing revisions. However, their scope often falls short when it comes to downstream data layers, such as as-built and as-maintained information.
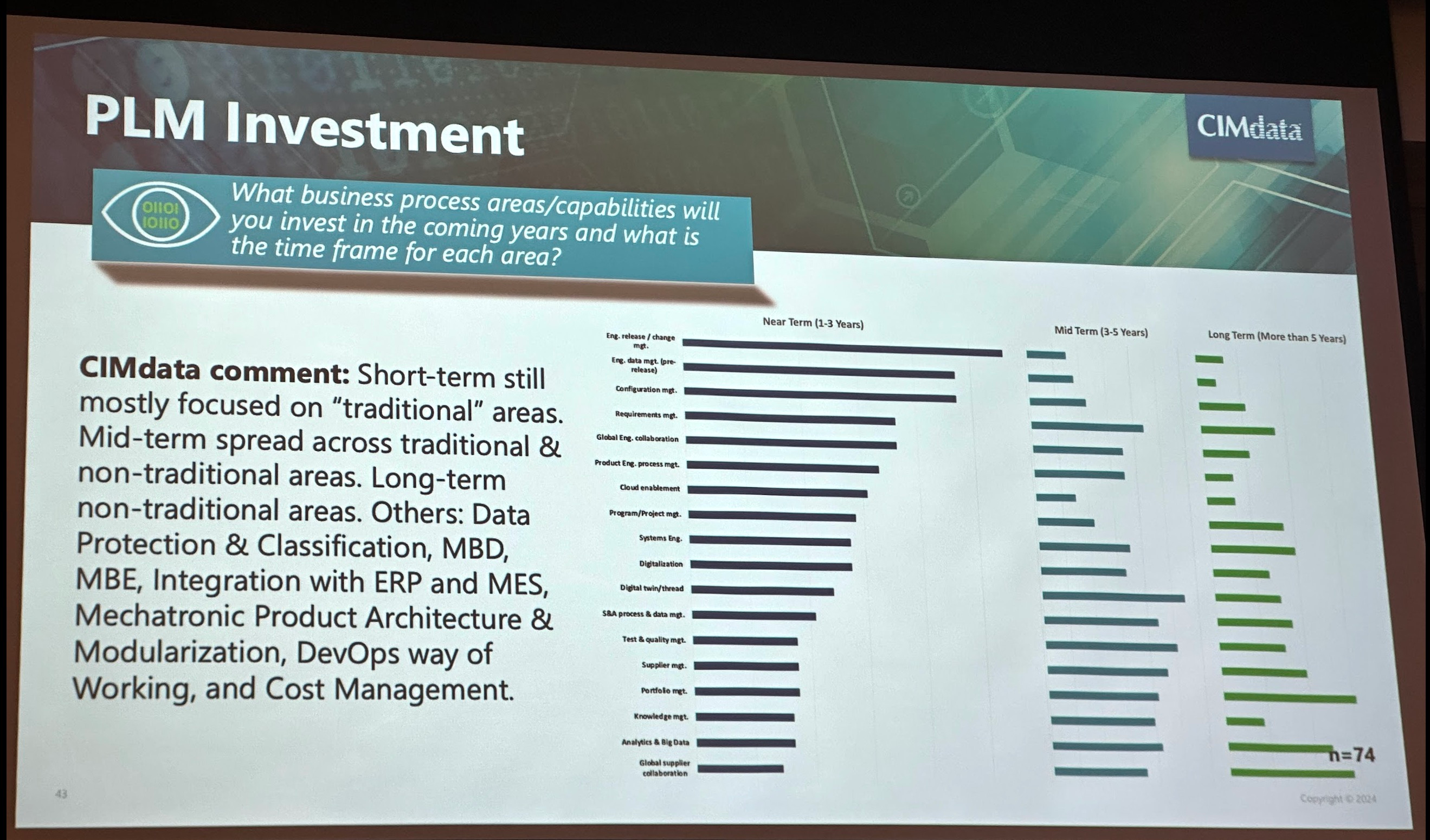
According to the last CIMdata research I captured a few weeks ago at CIMdata Industry and Market Forum in Ann Arbor, although the short term PLM investment is still targeting engineering release and change management, you can see an increased investment in digital twin that indicates potential ability to manage a digital copy of the product in each lifecycle stages. Check more in my article about Digital twin and lifecycle performance summarizing what I learned from recent CIMdata researches..
Barriers to Deploying Comprehensive Data Management Systems
When it comes to the question why companies are failing to deploy and establish robust data systems capable to handle multiple data layers effectively, the PLM strategy is usually the one that first to blame. Companies need to have a solid strategy for why to deploy sophisticated data management systems and business benefits. I agree, companies are lacking of digital strategy and proper data management infrastructure. Here are three main contributors to poor data management practices: (1) outdated data management architecture; (2) poor data traceability between systems, and (3) organizational silos both inside a company and with their suppliers, maintenance organizations and customers. In many examples, I’ve seen how lack of data management systems capable to manage data handover between lifecycle stages is really preventing companies from their ability to trace every bit of the information about products after it shipped from the manufacturing floor.
Strategies for Enhancing Downstream Data Layers
The associated risks not to manage proper product development lifecycle which includes data traced across the entire product development process from engineering bill of materials to manufacturing bill of materials and later outside of engineering department to manufacturing and then to “as maintained BOM” are huge. While companies are focusing on computer aided design (CAD) and engineering product definition, when it comes to manage physical product data and complete visibility about what is happening after the product is shipped is lacking.
The recent growing adoption of cloud systems should solve the problem according to many analysts and strategists, but even PLM systems are deployed, the “cloudless” is still only solve the problem of PLM installation and maintenance. From every other standpoint, these systems is a cloud hosted replica of existing PLM platforms. Here are three elements of PLM data management architecture that can make a difference for companies looking for management of multiple data representations (as engineering bill, as manufacturing bill and as maintained BOM).
Cloud-Based Infrastructure:
Embracing cloud-based infrastructure facilitates connected systems, enabling seamless collaboration and data sharing across departments and locations can help tremendously to create new types of systems connecting companies developing products with the customers and maintenance services and department organizations. However, to make it work, cloud systems must stop be “islands” of data. The data openness and connectivity will be extremely important.
Multi-Tenant Systems:
Until now, majority of PLM data architecture is single tenant. The logical database represents the ability of a company to manage a product structure, but then other companies working on maintenance of products are not really connected. The ability of maintenance organization or customers to get to the same system with multiple BOM type representation to see “only data” that is related to the maintenance while connected to original engineering representation can be a big deal. By adopting multi-tenant systems, companies can break down data silos and foster collaboration among multiple stakeholders. This approach promotes efficiency and scalability while minimizing redundancy.
Flexible Data Models:
PLM data management was developed for the last 20+ years, but basic data architectures remained unchanged. SQL databases is a core foundation of all PLM platforms today. At the same time, the data needed to maintain efficient data representation of multiple lifecycle twin including multiple BOM types is much more complex than needed for EBOM or event dual EBOM/MBOM solution. I recommend you to check my earlier articles discussing why and how future product data architectures will be using graph model to build digital product model. These new models offer scalability, agility, and a holistic view of product data throughout its lifecycle.
What is my conclusion?
The growing complexity of modern products necessitates a holistic approach to data management. While PLM systems have traditionally focused on engineering aspects, it’s crucial for companies to broaden their scope to encompass downstream data layers, particularly in maintenance and as-built information.
The key question manufacturing companeis are facing is how to embracing holistic data management to cover multiple lifecycle states – engineering, manufacturing planning, product builds and maintenance. Although it sounds like just a duplicated structure, the complexity of these data representations, complex identifications and change management requires rethink the way future PLM platforms should be built.
For manufacturing companies dealing with products with extensive lifecycles and maintenance requirements, prioritizing comprehensive data management is paramount. Moreover, as the complexity of products continues to increase, so does the importance of managing data throughout every step of the lifecycle.
In essence, navigating the complex web of PLM data layers requires foresight, adaptability, and a commitment to embracing technological advancements. By doing so, companies can streamline operations, enhance product quality, and stay ahead in an ever-evolving marketplace.
Just my thoughts…
Best, Oleg
Disclaimer: I’m co-founder and CEO of OpenBOM developing a digital-thread platform with cloud-native PDM & PLM capabilities to manage product data lifecycle and connect manufacturers, construction companies, and their supply chain networks. My opinion can be unintentionally biased.