
An interest in AI is spiking. ChatGPT touched the nerve of many people and it is on the mind of many people I’m talking to. The amount of news and social media posts that are flowing around about Generative AI, GPT-3, and ChatGPT is skyrocketing, which makes many of us think about how these new technologies and models can help us in our everyday life and businesses. A simple Google Trends check shows you what is happening.
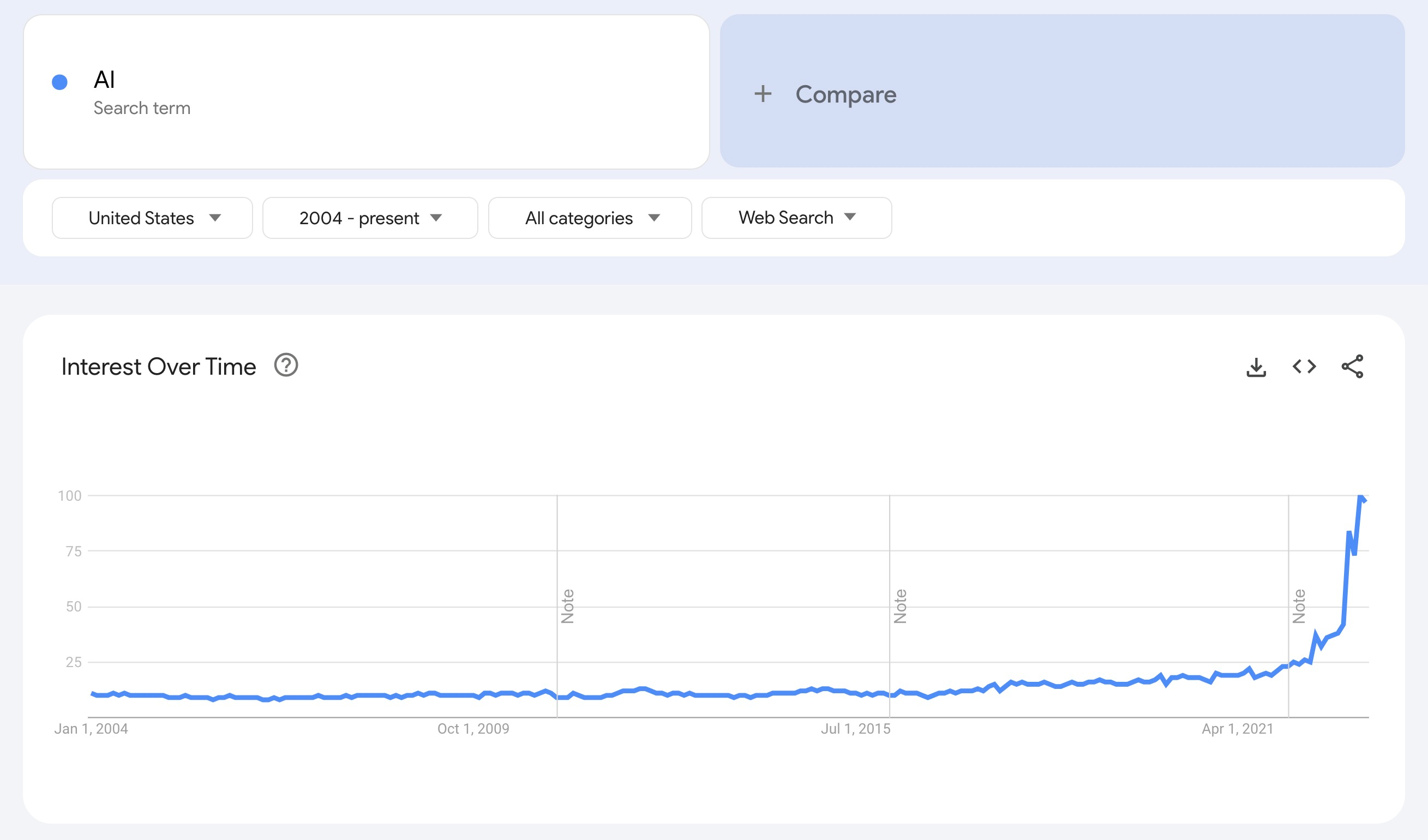
Let’s check AI progress with different applications – consumer, business and come to talk about industrial use cases.
How does AI change our everyday life?
What is clear is that GPT systems have something valuable and they can be used also in the everyday life. For example, I found myself using ChatGPT to create summaries, translate texts, and improve my web search experience. If you missed that capability, check Bing + ChatGPT features. For those that are preferring Google, don’t worry, because Google is planning to come with their version of Google Bard AI. If you didn’t check it, a good review is here
Low-Hanging Business Use Cases – Content and Marketing?
Let’s move into business use cases. ChatGPT and generative AI is making big waves in businesses that involved the content creation process. Automate content creation, making systems automatically generate ads, create articles, summaries, reviews, etc. The debates are everywhere with examples of successes and failures. I recommend you check the recording from GenAI virtual conferences, which can give you an interesting perspective on AI applications in marketing and some other business fields. I have personal experience with Jasper.AI, which in my view can be useful in some marketing and content-writing tasks. But I cannot see it going autonomous without being checked by humans. The same can be said about ChatGPT which easily can “hallucinate” about something that is far from reality.
AI and Industrial Use Cases
There are tons of conversations about AI usage in the industrial space. While you can easily see how the marketing software vendors will be placing “AI” stickers on everything now, I’d like to focus on tasks and system capabilities that are focusing on helping businesses to augment AI capabilities to improve the cognitive capabilities of knowledge workers with the ability to analyze information, analyzing impact and making a selection of options that beyond human intelligence and capabilities.
I’d like to refer you to a good publication from McKinsey & Company – The future is now: Unlocking the promise of AI in industrials. Check it out, I found it interesting. My favorite passage is related to the data (the topic I’m passionate about for years) and what role data will play in building future AI-driven engineering systems. Here is the passage:
After decades of collecting information, companies are often data rich but insights poor, making it almost impossible to navigate the millions of records of structured and unstructured data to find relevant information. This challenge is particularly important when engineers are troubleshooting new issues on highly complex systems (for example, aircraft, spacecraft, and power plants) to find the most relevant procedures, machine performance data, history of operations, and instances of relevant issues on similar subsystems. Engineers are often left relying on their previous experience, talking to other experts, and searching through piles of data to find relevant information. For critical issues, this high-stakes scavenger hunt is stressful at best and often leads to suboptimal outcomes.
The five use cases articles speak about make sense to me and I can see how companies and vendors can explore them for business values to improve business processes. The two use cases that resonated are (1) Knowledge Discovery and (2) Design Optimization.
Building knowledge graphs is an interesting space. I wrote multiple articles about Knowledge Graph on Beyond PLM. Check them out. The knowledge graph-based solutions can be super helpful in many analytical tasks related to the improvement of business processes such as change management, maintenance optimization, technical support, and many others.
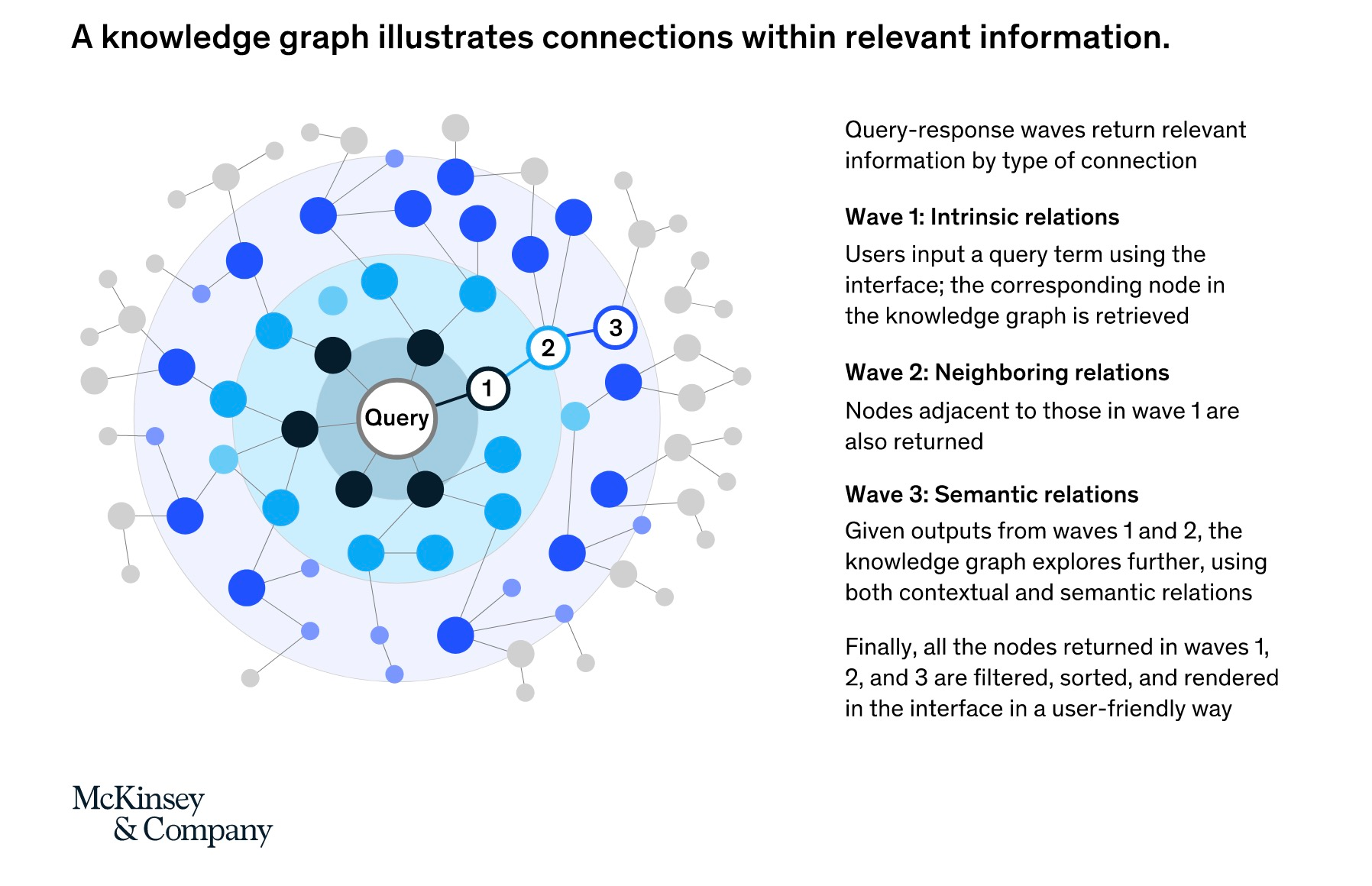
Another favorite example is related to BOM visualization and analysis of part usage. Digital Twin is another trending development and companies are looking at how to empower their design capabilities by bringing the analysis of the massive amounts of data into regular design tasks. The picture below shows how BOM visualization and analysis can be used to optimize part selection.
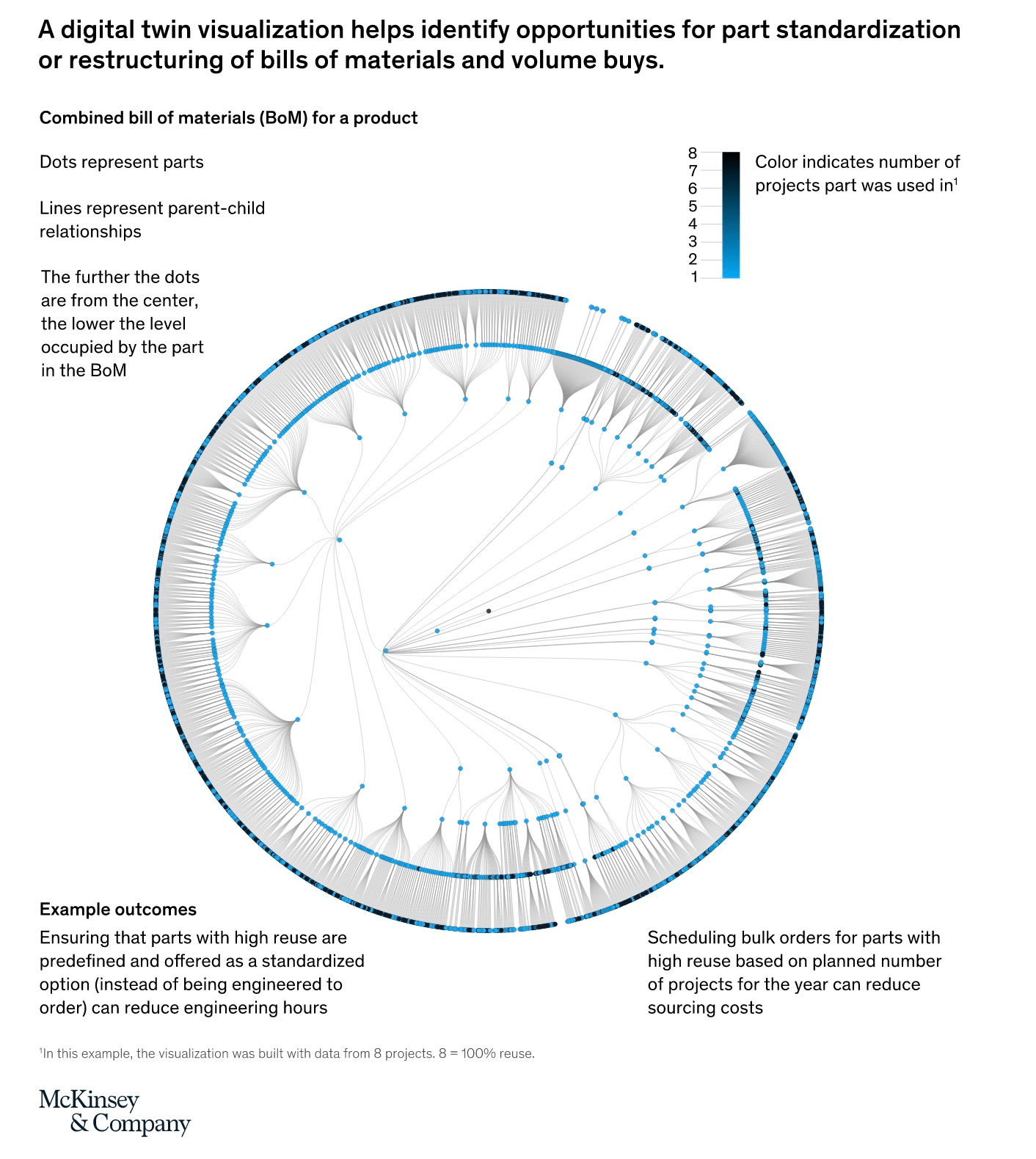
AI capabilities in PLM solution?
With the progress of AI technologies and applications in different fields, the question of how PLM vendors will be able to realize it for their own advantages is coming. Data was hoarded by PLM systems for years. Those systems represent a big part of engineering and manufacturing data history of operations, changes, product information, and many other pieces of data. Getting these data into use by AI systems is one of the first questions. However, adding the data that is sitting in thousands of legacy databases and zillions of Excel is even more important.
Here are 3 use cases I think can be interesting for PLM vendors.
- Impact analysis: capabilities to analyze the impact of changes, finding information dependencies and connections between systems, components, requirements, and functions can be very powerful. System design for complex products and change management functions can be significantly improved.
- Design Copilots: helping end users with manual tasks, to automate data creations and systems configurations can be very attractive. Imagine automation of top-down product design. Educational use cases can be also very relevant for these co-pilots.
- BOM analytics: bringing intelligence into BOM functions can be a superpower. Cost, supply chain, regulatory risks, and many other analyses can be much more powerful with AI.
What is my conclusion?
The time is now to discover AI technologies and find capabilities that can gradually improve existing PLM solutions and capture important use cases in the industrial world. At the same time, to avoid overhyping and bringing misunderstanding in the same way it happened to “digital transformation” projects with many of them leaving companies frustrated. The most important transformation in the minds of people responsible for enterprise and industrial solutions is to turn “data” from assets to business (will talk about it in my coming articles). AI needs data and without data, it won’t be as valuable as it could be. Just my thoughts…
Best, Oleg
Disclaimer: I’m co-founder and CEO of OpenBOM developing a global digital thread platform providing PDM, PLM, and ERP capabilities and new experience to manage product data and connect manufacturers, construction companies, and their supply chain networks. My opinion can be unintentionally biased.